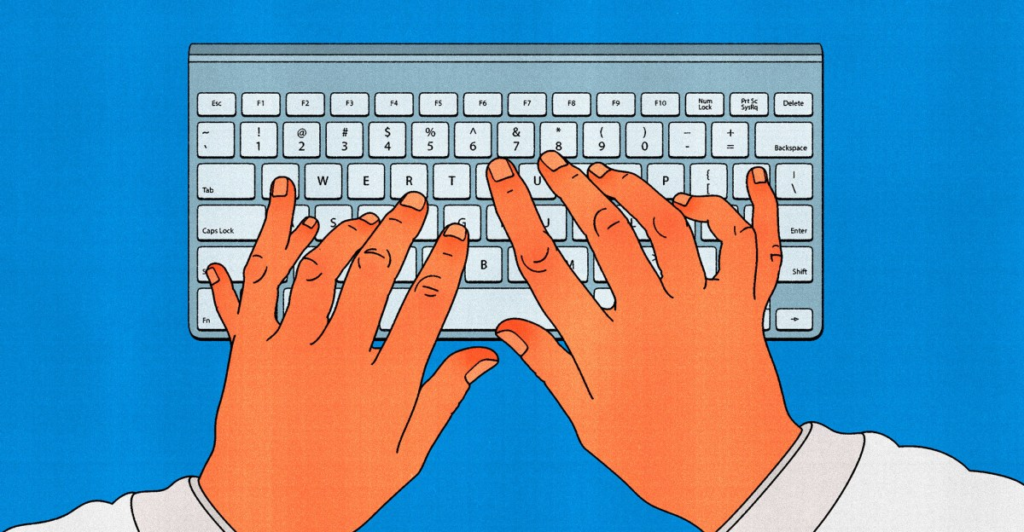
Big tech giants such as Microsoft, Google, and OpenAI are leading the charge in making AI chatbot technology more accessible to the general public, a tool that was once limited to research labs and experimental stages. Microsoft’s integration of AI into their Copilot product, Google’s launch of the Gemini AI, and OpenAI’s release of GPT-4 have brought large language models (LLMs) into the mainstream. These advancements are reshaping how we interact with technology, offering users everything from enhanced productivity tools to conversational agents capable of answering questions and generating content.
But what exactly powers these advanced AI systems, and how do they work? At the core of LLMs is a technology based on the principle of prediction. OpenAI’s GPT-3, for instance, explains that AI language models function through “a series of autocomplete-like programs that learn language” by analyzing “the statistical properties of the language.” Essentially, these models analyze vast amounts of text to identify patterns and relationships between words. This allows the AI to make “educated guesses” about what comes next in a sequence of words, much like how autocomplete on your phone or computer predicts the next word in your text messages.
However, these models don’t have access to a curated database of factual information or a deep understanding of the world. They rely on patterns and probabilities, making them adept at generating human-like text, but not necessarily accurate text. As James Vincent succinctly puts it, “These AI tools are vast autocomplete systems, trained to predict which word follows the next in any given sentence.” This means that LLMs can sound incredibly convincing, but they don’t guarantee factual accuracy. While they can create responses that sound plausible, this doesn’t mean they’re based on verifiable facts. In many cases, AI models can present false information confidently, often blending truth with fiction in ways that are hard to spot at first glance.
This raises important questions about the role of AI in generating and disseminating knowledge. While these systems are incredibly powerful and versatile, they also come with limitations. One of the biggest challenges in developing and deploying LLMs is ensuring they can be trusted to provide reliable and factually correct information. Since they’re not grounded in a database of factual knowledge, but rather trained to produce coherent responses, they sometimes generate misinformation or fail to provide nuanced answers to complex queries.
The rapid growth of the AI landscape adds to the complexity. As we’ve seen with products like Bing and Bard, the names of these AI tools have often changed as companies refine their offerings. These rebrands reflect the dynamic nature of the AI industry, where technologies are evolving at an unprecedented pace. What we once called search assistants or chatbots are now integral parts of broader technological ecosystems that influence everything from how we work to how we access information online.
Moreover, the capabilities of AI continue to expand. It’s not just about language anymore. With the emergence of multimodal models, such as OpenAI’s GPT-4, AI can now process text, images, and even video, making it capable of performing a broader range of tasks. AI tools are no longer limited to simple conversations; they can help generate written content, solve problems, assist with creative tasks, and even perform analyses across different types of media. As these tools become more integrated into daily life, from writing assistance to customer service, it’s clear that AI will play an increasingly prominent role in how we interact with information and technology.
There’s also the growing concern over the ethical implications of these tools. As AI becomes more capable, it raises important questions about privacy, bias, and accountability. Since LLMs are trained on massive datasets sourced from the internet, they can inadvertently learn and perpetuate harmful biases present in the data. This means that while AI may be excellent at generating text, it may also reflect and amplify societal biases, which can have real-world consequences, particularly when these models are deployed in high-stakes settings such as hiring, law enforcement, and healthcare.
Furthermore, the use of AI in generating content and automating tasks could also have economic and social implications. As AI becomes a tool for productivity, it raises questions about job displacement and how industries will adapt to an increasingly automated workforce. There are concerns that the widespread adoption of AI tools could lead to greater inequality, with some sectors benefiting immensely from automation while others may struggle to keep up.
As AI continues to evolve, the landscape will undoubtedly keep changing. New tools and applications will emerge, existing systems will become more refined, and regulatory frameworks will begin to take shape. The possibilities seem endless, but so do the challenges. Whether you’re using an AI chatbot for casual conversation, writing assistance, or problem-solving, it’s essential to remain aware of the technology’s limitations, its potential for error, and the larger societal impacts that will come with its widespread adoption.
With so much happening in the AI space, it’s clear that we’re only scratching the surface of what’s to come. From GPT-4 to Gemini and beyond, the evolution of these technologies promises to reshape the internet, how we communicate, and how we understand the world around us. As these tools continue to improve and become more ingrained in our daily lives, we can expect to see both exciting innovations and critical debates surrounding their use. The journey is just beginning, and you can be sure to witness all of it unfold in real time.